|
|
 |
 |

Mining in Textual Mountains
What if your computer could help you to look at mountains of documents the way a geologist looks at mountains of stone? You'd see past the rugged surface topography of peaks and valleys into the underlying strata of information. Like the geologist, you'd see your textual mountains in a new way. Best of all, you might find a good place to dig for gold.
Marti Hearst, Assistant Professor at the School of Information Management and Systems ("SIMS") of the University of California at Berkeley is looking for ways to prospect for nuggets of new knowledge in the mountains of text which have become accessible to computer based research thanks to the information and internetworking revolution. She calls this nascent field of inquiry "Text Data Mining" or TDM. Hearst says "it doesn't exist yet, but there's plenty of interest in getting it started."
Mining is not Retrieval
"When people hear about text data mining they often think it's intended as a way to make information easier to find on the Web," says Hearst. However, TDM does not refer to the familiar process of using keywords to search the Web for relevant pages. That process comes under the domain of Information Retrieval ("IR").
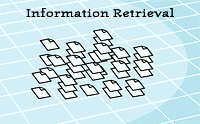
Information Retrieval
(IR) Selection and rejection of existing documents based on query
keywords or similar criteria.
|
|
|
|
Information retrieval is the process of finding information that is already known and has been inserted into a document by an author. In an IR search of the Web, a person wants to find relevant documents among all the other documents available online. Hearst describes information retrieval as a way to pull out the documents you are interested in and push away the others.
In contrast, text data mining is a way to examine a collection of documents and discover information not contained in any individual document in the collection. Hearst emphasizes this distinction because it's what makes text data mining so exciting. With TDM, the researcher seeks new information that wasn't previously known to anyone. Marti Hearst says, "Text data mining has the potential to be something much more, and something somewhat different than information retrieval."
In text data mining the researcher seeks relationships between the content of multiple texts and then sets about linking this information together to form a testable hypothesis about new information. The literature of medical research is a promising target for text data mining: a large and growing database of medical journal articles exists in digital format, and the formalized and detailed content delivery style of medical journal articles makes them a good subject for computerized TDM analysis. Because of the large number of journal articles published, it's unlikely that any one researcher could read (and remember) the contents of all of them. In theory, at least, TDM ought to be able to help researchers find possible linkages in published research findings, even across disciplines.
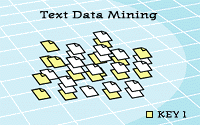
In Text Data Mining (TDM),
relationships between documents can generate new facts, not previously known.
|
|
|
|
Toward Text Data Mining
Researching medical journals for new hypotheses of cause and effect for a disease is an ideal case of what text data mining ought to be able to do. Don Swanson of the University of Chicago has already shown this can work in limited cases. But Marti Hearst says there are intermediary stages between information retrieval and full-blown TDM that are being worked on now. These include:
- Cut and Paste Tools to help a researcher create summaries of material from multiple sources.
- Document Intersection Finders to help a researcher find where multiple documents (of a generally divergent content) have passages dealing with the same content.
- Question Answering Tools based on semantic parsing of documents.
Hearst sees the development of question answering tools as one of the immediate challenges for the intermediate stages in the development of text data mining. The key area where progress is needed is the automation of natural language comprehension. Hearst says syntactic parsing of documents isn't enough, semantic parsing is needed.
For example, a semantic parsing task would be delving into a multi-disciplinary collection of journal articles to divide up sentences about epileptic seizures related to migraine occurrence. Hearst offers this synopsis of Don Swanson's work: "You might represent epileptic seizures as a symptom and magnesium as a kind of chemical or nutrient, then represent some relationship between the two - kind of a high level semantic representation. If you had that kind of a representation then you could ask the system questions like 'What sorts of effects does magnesium have on different systems of the body?' and it could reply 'it's deficiency can play a role in epileptic seizures.' So, we hope we'll have some kind of a question and answer system before we have the full information discovery system."
Next » Mining Tools.
Copyright © 1999, 2000 media.org.
|
 |