
 |

In The Stars

|
 |
|
Starry Night, by Martin Wattenberg, Mark Tribe and Alex Galloway uses an astronomy metaphor to visualize relationships between data. Wattenberg is also known for his work on SmartMoney's Map of the Market, which visually simplifies complex and time-sensitive financial information.
|
|
 |
|
 |
|
Mining Messages
Applications: Astronomy to Etymology and Beyond
While natural language understanding poses a special problem for text data mining, there are some parallels with number-based data mining. Hearst says, "Other data mining has really been discovering patterns across databases. So it's just like classification. In traditional classification you have situations like measurements in astronomy. You want to find out, 'is that a star or planet, or just some noise?' and so you run classification algorithms to try to get rid of the noise and see what the object is."
These applications are similar to the clustering and thematic overview extraction methods discussed above. "But," says Hearst, "there's an unexplored region in data mining as well as in text data mining, which is trying to discover new information from the records that are there. Facts. New facts that you could put in the database, as opposed to a rule that generally characterizes the data."
"I went to a workshop on the potential for link analysis," says Hearst. "One group, headed by John Picarelli reported on their efforts to track money laundering." It's a high-tech approach, but the strategies employed look a lot like old-fashioned gumshoe work.
"They have heuristics," says Hearst. "They look for people's relatives. They look for people that have gone out of the country. Various things they know have worked in the past." But, to mine text one needs to go to textual mountains, and money launderers try to keep their documentation on the QT.
Anywhere that one finds textual mountains, one may find text data mining opportunities, and some of these mines are beginning to disgorge some surprising discoveries. Marti Hearst says her team is interested in using text data mining to investigate the evolution of art, and the evolution of new media from a historical perspective. She believes this kind of research will come into its own as JSTOR and other similar services place larger collections of older material online.
For example, it's been used for etymological research. "The Oxford English Dictionary is supposed to include the oldest use of all these words," says Hearst. But using TDM techniques people are finding much earlier uses of expressions and words than had been previously believed. Hearst says grammarians have proscribed the use of "hopefully" to modify a sentence. So it's considered incorrect to say "Hopefully, I'll win the lottery today." Hearst says etymological research is calling that into question. The New York Times reported that Fred Shapiro, associate librarian at Yale Law School, used JSTOR to find occurrences of "hopefully" used to modify a whole sentence going back to 1851.
Getting the Message
"The government - NIST (National Institute of Standards and Technology) and DARPA (Defense Advanced Research Projects Agency) in particular - sponsors a series of competitions for people doing text analysis," says Hearst. "They held a message understanding contest." DARPA contacted several groups conducting natural language processing and IR research, and invited them to compete. In one recent competition, the evaluators marked up a collection of financial articles relating to mergers and acquisitions to create a test-bed for evaluating the effectiveness of various natural language understanding programs. The contestants were challenged to apply their natural language processing tools to the test-bed document collection and answer a question "who merged with (or was acquired by) whom" and "who was the CEO of each company". "This can be tricky because the proper names, people's names and company names often look similar, and so one of the sub-tasks is trying to pull out people's names and company names."
The program first makes a 'yes|no' decision; "Is this document about whether there has been a merger or acquisition?" If the answer is yes then the program goes through again and determines the answer to the merger/acquisition question. "Nobody gets 100%," says Hearst, "But real progress is being made."
The Future of Text Data Mining
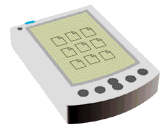
Still an academic pursuit, TDM has great potential for everyday applications -- but it may be a while before you see them on your PDA.
|
|
|
|
When will TDM move out of the research lab and into everyday use? Marti Hearst says she hopes to see real TDM in the next two to three years, "a long time in computer science these days, but we also have shorter-term goals."
"There are a lot of challenges. It's a very ambitious project. It's a risk filled project too." The difficulties are inherent in any project that relies upon objectifying and automating that quintessentially human skill - language interpretation. "I think we have some hope of overcoming these challenges that we didn't before because in the past we tried to construct models of the knowledge by hand and write a lot of the inference rules by hand. Now we have the advantage of a lot of statistical techniques, large text collections, and fast computers and big disks. All of these allow us to try to use statistical models to find associations between terms or to do things like semantic parsing that were too difficult to do before. The challenge before us is to create a new way to process and understand text."
Hearst believes this problem can be overcome by creating a good interface between an expert user and powerful computing resources. "We're focusing on having a user operating the system and not trying to automate everything." So the system will help the expert user, and the expert user will help the system. But, Hearst says language interpretation is not a trivial problem. "Don't believe the hype. There's a lot of hype about people doing things, and anything about language processing, and any claims about being able to process language I'd say be very skeptical of. And of fancier retrieval methods, I'd say, be very skeptical."
Copyright © 1999, 2000 media.org.
|